Minimum Risk Equivariant Estimators of Percentiles in Locationã‚âscale Families of Distributions
1. Introduction
The semiparametric moment status models are divers through estimating equations
where denotes the mathematical expectation,
is a random vector,
is the unknown true value of the parameter of interest which is assumed to exist unique, and is some specified measurable -valued role defined on . Such models are pop in statistics and econometrics, see eastward.chiliad., Qin and Lawless (1994), Haberman (1984), Sheehy (1987), McCullagh and Nelder (1983), Owen (2001) and the references therein. Denoting
the probability distribution of the random vector
, then the above estimating equations can be written as
Let exist the collection of all signed finite measures (south.f.m.) on the Borel -field such that . The submodel , associated to a given value , consists of all s.f.m.'s satisfying linear constraints induced by the vector valued function , namely,
with . The statistical model which we consider can be written equally
Let be an i.i.d. sample of the random vector with unknown probability distribution . The problems of testing the model , confidence region and point estimations of , take been widely investigated in the literature. Hansen (1982) considered generalized method of moments (GMM) in order to guess . Hansen et al. (1996) introduced the continuous updating (CU) estimate. Asymptotic confidence regions for the parameter accept been obtained past Owen (1988) and Owen (1990), introducing the empirical likelihood (EL) arroyo. It has been used, in the context of model (1), by Qin and Lawless (1994) and Imbens (1997) introducing the EL estimate for the parameter . The recent literature in econometrics focusses on such models; Smith (1997), Newey and Smith (2004) provided a class of estimates called generalized empirical likelihood (GEL) estimates which contains the EL and the CU ones. Among other results pertaining to EL, Newey and Smith (2004) stated that EL estimate enjoys asymptotic optimality properties in term of efficiency when bias corrected among all GEL estimates including the GMM i. Broniatowski and Keziou (2012) proposed a general arroyo through empirical divergences and duality technique which includes the above methods in the general context of signed finite measures under moment condition models (ane
). These approach allows the asymptotic study of the estimates and associated test statistics both under the model and under misspecification, leading to new results, in particular, for the EL approach. Note that all the proposed estimates including the EL i are by and large biased, and that the problem of their finite sample efficiency, at our knowledge, accept not yet been studied.
The aim of the present paper is to investigate the finite-sample optimality holding interpretation in the context of semiparametric model (1). We volition talk over the trouble of constructing minimum take a chance equivariant estimates (MRE) for the parameter , as well as the trouble of the numerical calculation of these estimates.
We recall in the following lines, for the above estimation problem, the notions of group transformations on the random vector space, model invariance and the induced group of transformations on the parameter space, loss invariance and equivariance interpretation; nosotros refer to the unpublished preprint of Hoff (2012) for an excellent presentation of the in a higher place notions, and the book of Lehmann and Casella (1998).
Allow be a collection, of ane-to-one transformations from the vector space in , which we assume to be a "group", in the sense that, information technology should be closed nether both composition and inversion, namely,
The group tin be extended to a group of transformations on the sample space, onto , which will exist denoted , every bit follows
Nosotros will consider ii kinds of transformation groups,
-
"condiment"
where is some subset of ,
-
"multiplicative"
where is diagonal matrix, with entries or with possibly some entries equal to one.
We presume that the model given in (ane) is invariant nether the considered group of transformations , in the sense that,
The induced grouping of transformations on the parameter space, onto , denoted hereafter, will be defined as follows. Let be any transformation belonging to , and consider any random vector such that . Then, by identifiability supposition, there exists a unique such that . Past invariance assumption (4), of the model to the group , the distribution belongs to . Therefore, there exists a unique (past indentifiability) such that . Denote then past the bijection induced by on the parameter space onto , divers past
The induced group on the parameter space, onto , is and so defined to exist
Two points are said equivalent iff for some The orbit , of a point , is defined to be the set of equivalent points:
Nosotros volition assume that there is only 1 orbit of , i.e.,
which means that the group of transformation is rich plenty allowing to become from any signal in to some other via some transformation . In such case, the group is said to exist "transitive" over .
We requite hither some examples for analogy. In all the examples beneath, nosotros can see that the group is transitive over .
Case 1 .
Sometimes we accept data relating the commencement and 2nd moments of a random variable
(see e.g. Godambe and Thompson (1989) and McCullagh and Nelder (1983)). Permit be an i.i.d. sample of a random variable with mean , and presume that , where is a known function. Our aim is to estimate . The data about the distribution of can be expressed in the form of (1) past taking If we have the parameter space to be , then it is straightforward to run across that the model is invariant to the condiment grouping of transformations
if for some , and invariant to the multiplicative group
if for some .The induced groups on the parameter space are, respectively,
and
Instance 2 .
Permit be an i.i.d. sample of a bivariate random vector with . In this example, nosotros tin can take If we consider , and then the model is invariant with respect to the groups
or
The induced groups on are, respectively,
and
A some what similar problem is when is known, and is to be estimated, by taking Such bug are common in survey sampling (run into e.g. Kuk and Mak (1989) and Chen and Qin (1993)). Taking , the model is and so invariant with respect to the groups
or
The induced groups on are, respectively,
and
Case 3 .
Permit be an i.i.d. sample of a random variable with distribution such that , where , . The known intervals may exist bounded or unbounded, and are known nonnegative numbers. The data well-nigh tin be written under the course of model (1) taking and . The model in this case is invariant to the groups
or
and the induced groups on the parameter infinite are, respectively,
and
Example iv .
Allow be an i.i.d. sample of a random variable with continuous distribution such that , and , where is known and is to be estimated. Note that
is the quantile of social club
of the variable
, and that the variance of
is assumed to be known and equal to one. This problem can be written nether the grade of model (1) taking and . The model in this example is invariant with respect to the additive group
and the induced group on is
Example 5 .
Let be an i.i.d. sample of a random variable with continuous distribution such that and , where is known and is to exist estimated. Annotation that is the quantile of order of the variable . This trouble can be written under the form of model (1) taking and . The model in this case is invariant with respect to the multiplicative group
and the induced group on is
Example 6 .
Let be an i.i.d. sample of a random vector with continuous distribution such that , where is some specified measurable part, and is to be estimated. Nosotros can consider also the case where some components of are known and that the other components are to be estimated. It is clear that the corresponding model defined in (1), taking and , is invariant to the additive grouping
and the induced grouping on the parameter is
As well, if the data are such that , where is some specified measurable function, and is to exist estimated, then the corresponding model , taking , is invariant to the multiplicative group
and the induced grouping on the parameter is
In all the sequel, without loss of generality, we assume that the model and the grouping of transformation are such that
Annotation that this assumption implies the status (4) that the model is invariant under .
In all the following, when estimating by an guess
, nosotros consider the quadratic loss part
if the model is invariant with respect to additive group, and the loss function is taken to be relative quadratic
if the model is invariant with respect to the multiplicative group.
Definition 7 .
(invariant loss under a group of transformations). A loss office , where denotes the prepare of the parameter estimates (called decision space), is invariant nether a transformation iff for whatever estimate , there exists a unique such that Nosotros denote so by the bijection, from onto , such that . Hence, we have
We denote by the induced group on the decision space .
Definition 8 .
Assume that the estimation problem is invariant under the group . Let and be, respectively, the induced groups on the parameter space and the decision space . An estimate is said to exist equivariant iff
We will come across, under condition (6), that the empirical minimum divergence estimates, introduced in Broniatowski and Keziou (2012), are equivariant for the higher up models, using results on the existence and characterization of the distribution ont the sets . First, we call back the definition of -divergences and some of their backdrop. Let be a convex role from onto with , and such that its domain, is an interval, with endpoints satisfying
where is the Radon-Nikodym derivative of w.r.t. . When is not a.c.westward.r.t. , we set . For any probability distribution , the mapping is convex and takes nonnegative values. When then . Furthermore, if the function is strictly convex on a neighborhood of , then nosotros accept
All the above properties are presented in Csiszár (1963), Csiszár (1967) and in Chapter 1 of Liese and Vajda (1987), for divergences divers on the set of all probability distributions . When the -divergences are extended to , so the aforementioned arguments every bit developed on hold. When defined on , the Kullback-Leibler , modified Kullback-Leibler , , modified , Hellinger , and divergences are respectively associated to the convex functions , , , , and . All these divergences except the one, vest to the course of the and then-called power divergences introduced in Cressie and Read (1984) (see likewise Liese and Vajda (1987) and Pardo (2006)). They are divers through the grade of convex functions
if , and . And so, the divergence is associated to , the to , the to , the to and the Hellinger altitude to . We extend the definition of the ability divergences functions onto the whole fix of signed finite measures as follows. When the office is not defined on or when is defined on simply is not convex (for case if ), we extend the definition of as follows
Note that for -divergence, the respective function is convex and defined on whole . In this newspaper, for technical considerations, we assume that the functions are strictly convex on their domain , twice continuously differentiable on , the interior of their domain. Hence, , and for all , . Here, and are used to denote respectively the first and the second derivative functions of . Note that the higher up assumptions on are non restrictive, and that all the power functions , see (12), satisfy the above conditions, including all standard divergences.
ii. Minimum empirical divergence estimates
Let denote an i.i.d. sample of a random vector with probability distribution . Let be the associated empirical measure, namely,
where denotes the Dirac measure at betoken , for all . For a given , the "plug-in" approximate of is
If the projection of on exists, then information technology is clear that is a s.f.g. (or maybe a probability distribution) a.c.w.r.t. ; this ways that the support of must exist included in the gear up . So, define the set
which may be seen as a subset of . And then, the plug-in approximate (13) can exist written equally
In the same way,
can be estimated by
Past uniqueness of and since the infimum is reached in , we estimate through
The expression of the estimate , given in (xv), is the solution of a convex optimization problem under convex constrained subset in . In order to transform this problem to an unconstrained one, we will brand utilize of the Fenchel-Lengendre transform, denoted , of the convex role , every bit well as some other duality arguments. It is defined by
For convenience, we recall some backdrop of the convex conjugate of . For the proofs we tin can refer to Department 26 in Rockafellar (1970). Theses backdrop volition be used to determine the convex conjugates of some standard divergence functions ; encounter Tabular array 1 beneath. The function in turn is convex and closed, its domain is an interval with endpoints
satisfying with . Annotation that the interval
tin can be different from , the existent domain of given by (19). This holds when or is finite and or is finite, respectively. For example, for the convex function
we have and , and we can run across that the domain of the corresponding -role is which is unlike from The two intervals and coincide if the function is "essentially smooth", i.e., differentiable with
The strict convexity of on its domain is equivalent to the condition that its cohabit is essentially smooth, i.eastward., differentiable with
Conversely, is substantially smooth on its domain if and only if is strictly convex on its domain .
In all the sequel, we assume additionally that is essentially smooth. Hence, is strictly convex on its domain , and information technology holds that
and
where denotes the inverse of the derivative function of . It holds also that is twice continuously differentiable on with
In particular, and . Manifestly, since is assumed to be airtight, we take
which may be finite or space. Hence, past closedness of , likewise we have
Finally, the showtime and 2nd derivatives of in and are defined to be the limits of and when and when . The outset and second derivatives of in and are divers in a similar way. In Tabular array 1, using the above properties, we give the convex conjugates of some standard divergence functions , associated to standard divergences. We make up one's mind too their domains, respectively, and .
Using some duality arguments, see Broniatowski and Keziou (2012), we can show that, for whatever , if there exists in such that
and so
with dual attainment. Conversely, if there exists some dual optimal solution
such that
and so the equality (25) holds, and the unique optimal solution of the key problem
namely, the projection of on , is given past
where is solution of the system of equations
In view of the last results, using the notations
and
we obtain the following equivalent expressions to the estimates , and , see (thirteen), (sixteen) and (17),
and
We will show that for whatever divergence , the estimate is invariant with respect to loss for the additive group, and invariant with respect to loss for the multiplicative group. Offset, we betrayal the asymptotic counterpart of the estimates (29), (31) and (33). In particular, we requite results about existence and label of the projection of on the model . The characterization of the project will exist of not bad importance in calculating the minimum risk equivariant approximate. We accept; see Theorem i in Broniatowski and Keziou (2006):
Proposition 10 .
Let be a given value in . Assume that for all , and that there exists in such that and ane one 1 The strict inequalities mean that
Then, we have
with dual attainment. Conversely, if at that place exists a dual optimal solution
belonging to the interior (in ) of the set
then the dual equality (35) holds, and the unique optimal solution of the cardinal problem , namely, the project of on , is given past
where is the solution of the system of equations
Furthermore, the solution is unique if the functions are linearly contained in the sense that for all with
Suggestion 12 .
Presume that condition (vi) holds. And so, the minimum empirical -divergence estimates are equivariant
-
to the additive group of transformations with respect to the loss;
-
to the multiplicative group of transformations with respect to the loss.
Moreover, in both cases, the induced group of transformations on the space of estimates is equal to , the group of transformations on the parameter space , in the sense that
Corollary 13 .
For any estimate , the corresponding loss function is abiding.
In view of the higher up corollary, for the additive group, in order to obtain the uniform minimum risk estimate, we can compute the take chances of whatsoever gauge under the item value , and then select the estimate that minimizes the risk. Likewise, if a multiplicative group is considered, to obtain the uniform minimum risk judge, we tin can compute the risk of any guess under the particular value , and then select the gauge that minimizes the risk. To practice this, we will kickoff characterize the equivariant estimates.
Definition 14 .
A functional is "invariant" iff
Definition 15 .
A functional is a "maximal invariant" iff information technology is invariant and satisfies
Proposition 17 .
Assume that the estimation trouble is invariant nether the group . Let and be, respectively, the induced groups on the parameter space and the decision infinite . Let be any equivariant estimate. Then, an gauge is equivariant iff
for some invariant functional , i.due east.,
Suggestion eighteen .
(Hoff (2012), Theorem 3). A functional is invariant iff it is a role of a maximal invariant functional .
Combining the above results, we obtain
Proposition 19 .
Permit be any equivariant gauge. So is equivariant iff
where is some function of the maximal invariant functional
3. UMRE guess for additive group
Let be whatsoever i of the equivariant estimates , and presume that . Consider the loss. In view of the above statements, the UMRE judge of is then given past
where , and is the provisional expectation of given , under the assumption that . We requite in the following an asymptotic approximation to the conditional expectation
using the upshot of Jurečková and Picek (2009). Straightforward calculs, shows that the score function, of the semiparametric exponential family unit (38), can be written every bit
where and are, respectively, the derivative w.r.t. , of and the solution of the system (39). The derivative tin exist derived by the implicit function theorem. Denote with
Let
Then, by the implicit role theorem, nosotros have
Observe that, for true value , since , we obtain for the true value the more than simpler expression
Let
Under some integrability assumptions, past dominated convergence theorem, we obtain
which is the contrary of the Fisher information matrix.
Theorem 21 .
Under some regularity atmospheric condition, we accept
which gives the following approximation of the UMRE guess
where is the empirical estimate of the Fisher information matrix , given by
with
is the solution of the empirical version of the system (39), i.east., the solution in of
and is the gradient of at the point given by
where
and
4. UMRE estimate for multiplicative group
Permit be whatever one of the equivariant estimates of , and presume that . Consider the loss. In view of the above statements, the UMRE approximate of is given by
where , and is the conditional expectation given , under the assumption that .
five. Simulation results
Example 22 .
Consider the model
where . Let be a random variable with distribution with . The model is invariant to the additive group. We compare the mean square errors (MSE) of the EL approximate and the proposed UMRE approximate using the approximation (49), for the sample sizes , with runs. Nosotros tin run across, from effigy i, that the proposed estimate improves the EL ane for moderate sample sizes.
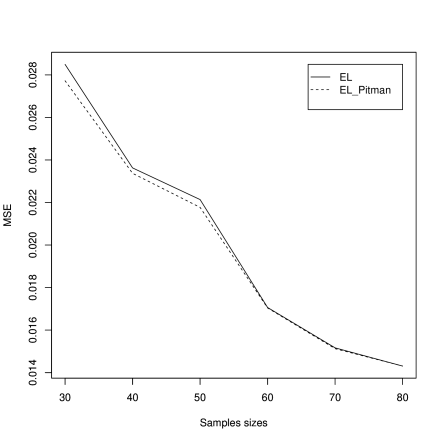
References
- Broniatowski and Keziou (2006) Broniatowski, M. and Keziou, A. (2006). Minimization of -divergences on sets of signed measures. Studia Sci. Math. Hungar.; arXiv:1003.5457, 43(4), 403–442.
- Broniatowski and Keziou (2012) Broniatowski, M. and Keziou, A. (2012). Divergences and duality for estimation and test under moment condition models. J. Statist. Plann. Inference, 142(9), 2554–2573.
- Chen and Qin (1993) Chen, J. H. and Qin, J. (1993). Empirical likelihood estimation for finite populations and the constructive usage of auxiliary information. Biometrika, 80(ane), 107–116.
- Cressie and Read (1984) Cressie, N. and Read, T. R. C. (1984). Multinomial goodness-of-fit tests. J. Roy. Statist. Soc. Ser. B, 46(iii), 440–464.
- Csiszár (1963) Csiszár, I. (1963). Eine informationstheoretische Ungleichung und ihre Anwendung auf den Beweis der Ergodizität von Markoffschen Ketten. Magyar Tud. Akad. Mat. Kutató Int. Közl., 8, 85–108.
- Csiszár (1967) Csiszár, I. (1967). On topology properties of -divergences. Studia Sci. Math. Hungar., 2, 329–339.
- Godambe and Thompson (1989) Godambe, 5. P. and Thompson, M. E. (1989). An extension of quasi-likelihood estimation. J. Statist. Plann. Inference, 22(2), 137–172. With word and a respond by the authors.
- Haberman (1984) Haberman, S. J. (1984). Aligning by minimum discriminant information. Ann. Statist., 12(3), 971–988.
- Hansen et al. (1996) Hansen, 50., Heaton, J., and Yaron, A. (1996). Finite-sample properties of some culling gmm estimators. Periodical of Business organisation and Economical Statistics, 14, 462–2800.
- Hansen (1982) Hansen, L. P. (1982). Large sample properties of generalized method of moments estimators. Econometrica, l(four), 1029–1054.
- Hoff (2012) Hoff, P. D. (2012). Equivariant estimation. Preprint.
- Imbens (1997) Imbens, Yard. W. (1997). One-footstep estimators for over-identified generalized method of moments models. Rev. Econom. Stud., 64(3), 359–383.
- Jurečková and Picek (2009) Jurečková, J. and Picek, J. (2009).
Minimum risk equivariant reckoner in linear regression model.
Statist. Decisions, 27(1), 37–54. - Kuk and Mak (1989) Kuk, A. Y. C. and Mak, T. Chiliad. (1989). Median interpretation in the presence of auxiliary information. J. Roy. Statist. Soc. Ser. B, 51(2), 261–269.
- Lehmann and Casella (1998) Lehmann, E. L. and Casella, G. (1998). Theory of point estimation. Springer Texts in Statistics. Springer-Verlag, New York, second edition.
- Liese and Vajda (1987) Liese, F. and Vajda, I. (1987). Convex statistical distances, book 95. BSB B. Grand. Teubner Verlagsgesellschaft, Leipzig.
- McCullagh and Nelder (1983) McCullagh, P. and Nelder, J. A. (1983). Generalized linear models. Monographs on Statistics and Applied Probability. Chapman & Hall, London.
- Newey and Smith (2004) Newey, West. Thousand. and Smith, R. J. (2004). Higher order properties of GMM and generalized empirical likelihood estimators. Econometrica, 72(1), 219–255.
- Owen (1990) Owen, A. (1990). Empirical likelihood ratio confidence regions. Ann. Statist., 18(1), 90–120.
- Owen (1988) Owen, A. B. (1988).
Empirical likelihood ratio conviction intervals for a single functional.
Biometrika, 75(two), 237–249. - Owen (2001) Owen, A. B. (2001). Empirical Likelihood. Chapman and Hall, New York.
- Pardo (2006) Pardo, Fifty. (2006). Statistical inference based on divergence measures, book 185 of Statistics: Textbooks and Monographs. Chapman & Hall/CRC, Boca Raton, FL.
- Qin and Lawless (1994) Qin, J. and Lawless, J. (1994). Empirical likelihood and general estimating equations. Ann. Statist., 22(1), 300–325.
- Rockafellar (1970) Rockafellar, R. T. (1970). Convex analysis. Princeton University Press, Princeton, N.J.
- Sheehy (1987) Sheehy, A. (1987). Kullback-Leibler constrained estimation of probability measures. Study, Dept. Statistics, Stanford Univ.
- Smith (1997) Smith, R. J. (1997). Alternative semi-parametric likelihood approches to generalized method of moments estimation. Economic Journal, 107, 503–519.
Source: https://deepai.org/publication/uniform-minimum-risk-equivariant-estimates-for-moment-condition-models
0 Response to "Minimum Risk Equivariant Estimators of Percentiles in Locationã‚âscale Families of Distributions"
Post a Comment